Enabling Active Learning Pedagogy and Insight Mining with a Grammar of Model Analysis
Contents
Example Notebook
This notebook implements many of the examples presented in the paper.
import grama as gr
import numpy as np
import pandas as pd
DF = gr.Intention()
gr.hide_traceback() # To simplify errors shown in Jupyter
%matplotlib inline
md_example = (
gr.Model("An example model")
# Overloaded `>>` provides pipe syntax
>> gr.cp_vec_function(
fun=lambda df: gr.df_make(f=df.x+df.y+df.z),
var=["x", "y", "z"],
out=["f"],
)
>> gr.cp_bounds(x=(-1, +1))
>> gr.cp_marginals(
y=gr.marg_mom("norm", mean=0, sd=1),
z=gr.marg_mom("uniform", mean=0, sd=1),
)
>> gr.cp_copula_gaussian(
df_corr=gr.df_make(var1="y", var2="z", corr=0.5)
)
)
The model representation presents a helpful summary:
md_example
/Users/zach/Git/py_grama/grama/marginals.py:336: RuntimeWarning: divide by zero encountered in double_scalars
model: An example model
inputs:
var_det:
x: [-1, 1]
var_rand:
y: (+0) norm, {'mean': '0.000e+00', 's.d.': '1.000e+00', 'COV': inf, 'skew.': 0.0, 'kurt.': 3.0}
z: (+0) uniform, {'mean': '0.000e+00', 's.d.': '1.000e+00', 'COV': inf, 'skew.': 0.0, 'kurt.': 1.8}
copula:
Gaussian copula with correlations:
var1 var2 corr
0 y z 0.5
functions:
f0: ['x', 'y', 'z'] -> ['f']
Construct a default parameter sweep
(
md_example
>> gr.ev_sinews(df_det="swp")
>> gr.pt_auto()
)
/Users/zach/opt/anaconda3/envs/evc/lib/python3.9/site-packages/plotnine/utils.py:371: FutureWarning: The frame.append method is deprecated and will be removed from pandas in a future version. Use pandas.concat instead.
Calling plot_sinew_outputs....
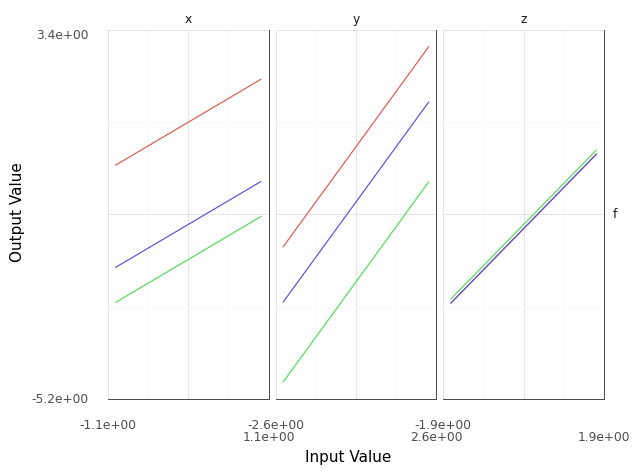
<ggplot: (8765340788697)>
Function Defaults¶
Most concise form: Evaluate a model with default arguments, construct an automatic plot.
p = (
md_example
>> gr.ev_sinews(df_det="swp") # Default parameters
>> gr.pt_auto() # Default visual
)
p.save("example-sweep.png")
Calling plot_sinew_outputs....
/Users/zach/opt/anaconda3/envs/evc/lib/python3.9/site-packages/plotnine/ggplot.py:719: PlotnineWarning: Saving 6.4 x 4.8 in image.
/Users/zach/opt/anaconda3/envs/evc/lib/python3.9/site-packages/plotnine/ggplot.py:722: PlotnineWarning: Filename: example-sweep.png
/Users/zach/opt/anaconda3/envs/evc/lib/python3.9/site-packages/plotnine/utils.py:371: FutureWarning: The frame.append method is deprecated and will be removed from pandas in a future version. Use pandas.concat instead.
Override the evaluation default to produce more sweeps.
(
md_example
## Override default parameters
>> gr.ev_sinews(df_det="swp", n_sweeps=10)
>> gr.pt_auto()
)
Calling plot_sinew_outputs....
/Users/zach/opt/anaconda3/envs/evc/lib/python3.9/site-packages/plotnine/utils.py:371: FutureWarning: The frame.append method is deprecated and will be removed from pandas in a future version. Use pandas.concat instead.
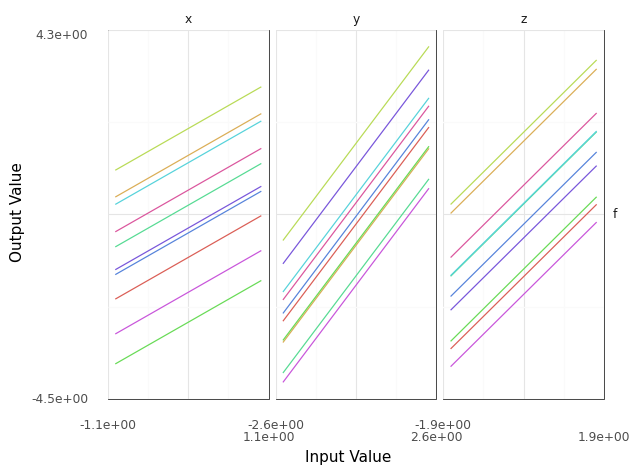
<ggplot: (8765323858170)>
Override the autoplot to construct a more targeted manual plot.
(
md_example
>> gr.ev_sinews(df_det="swp")
## Construct a manual plot
>> gr.tf_filter(DF.sweep_var == "x")
>> gr.ggplot(gr.aes("x", "f", group="sweep_ind"))
+ gr.geom_line()
)
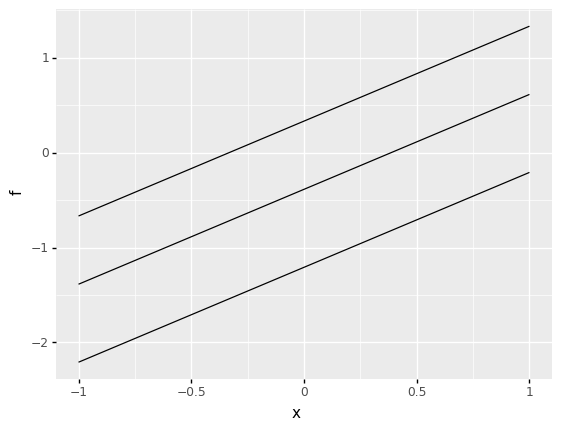
<ggplot: (8765332735501)>
Case Studies¶
1. Planned Errors as Teachable Moments¶
It is possible to define a grama model without a copula:
md_flawed = (
gr.Model("An example model")
>> gr.cp_vec_function(
fun=lambda df: gr.df_make(f=df.x+df.y+df.z),
var=["x", "y", "z"],
out=["f"],
)
>> gr.cp_bounds(x=(-1, +1))
>> gr.cp_marginals(
y=gr.marg_mom("norm", mean=0, sd=1),
z=gr.marg_mom("uniform", mean=0, sd=1),
)
)
However, this flawed model will throw an error when used in a probabilistic analysis:
(
md_flawed
>> gr.ev_sample(n=1000, df_det="nom")
)
ValueError:
Present model copula must be defined for sampling.
Use CopulaIndependence only when inputs can be guaranteed
independent. See the Documentation chapter on Random
Variable Modeling for more information.
https://py-grama.readthedocs.io/en/latest/source/rv_modeling.html
2. Encouraging Sound Analysis¶
A comparative example
Manual approach¶
## Manual coordination
# Model data
x_lo = -1; x_up = +1;
y_lo = -1; y_up = +1;
f_model = lambda x, y: x**2 * y
# Analysis parameters
nx = 10 # Grid resolution for x
y_const = [-1, 0, +1] # Constant values for y
# Generate data
data = np.zeros((nx * len(y_const), 3))
for i, x in enumerate(np.linspace(x_lo, x_up, num=nx)):
for j, y in enumerate(y_const):
data[i + j*nx, 0] = f_model(x, y)
data[i + j*nx, 1] = x
data[i + j*nx, 2] = y
# Package for visual
df_manual = pd.DataFrame(
data=data,
columns=["f", "x", "y"],
)
(
df_manual
>> gr.ggplot(gr.aes("x", "f", group="y", color="y"))
+ gr.geom_line()
)
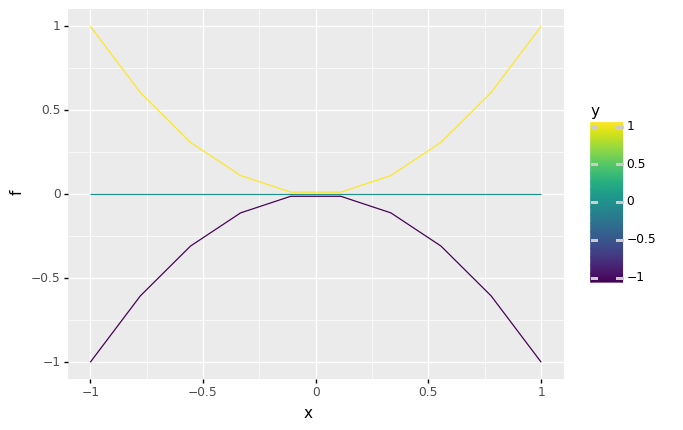
<ggplot: (8765362710010)>
Grama approach¶
## Grama approach
# Model data
md_gr = (
gr.Model()
>> gr.cp_vec_function(
fun=lambda df: gr.df_make(f=df.x**2 * df.y),
var=["x", "y"],
out=["f"],
)
>> gr.cp_bounds(
x=(-1, +1),
y=(-1, +1),
)
)
# Generate data
df_gr = gr.eval_sinews(
md_gr,
df_det="swp",
n_sweeps=3,
)
(
df_gr
>> gr.tf_filter(DF.sweep_var == "x")
>> gr.ggplot(gr.aes("x", "f", group="y", color="y"))
+ gr.geom_line()
)
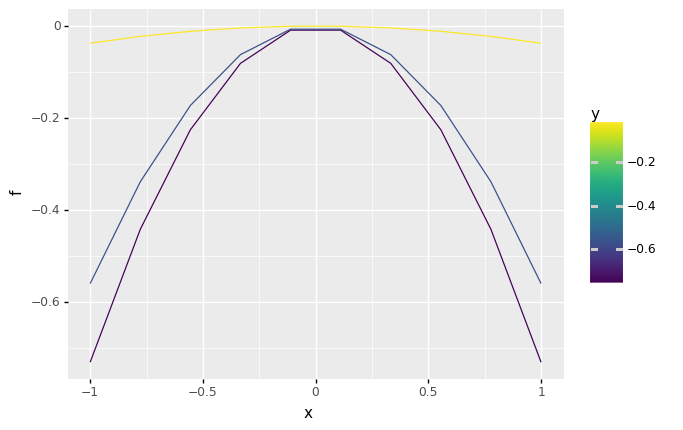
<ggplot: (8765332851008)>
In-the-wild Example¶
md_car = (
gr.Model("Accel Model")
>> gr.cp_function(
fun = calculate_finish_time,
var = ["GR", "dt_mass", "I_net" ],
out = ["finish_time"],
)
>> gr.cp_bounds(
GR=(+1,+4),
dt_mass=(+5,+15),
I_net=(+.2,+.3),
)
)
gr.plot_auto(
gr.eval_sinews(
md_car,
df_det="swp",
#skip=True,
n_density=20,
n_sweeps=5,
seed=101,
)
)
The following is the cropped form of the student parameter sweep, presented in the paper.
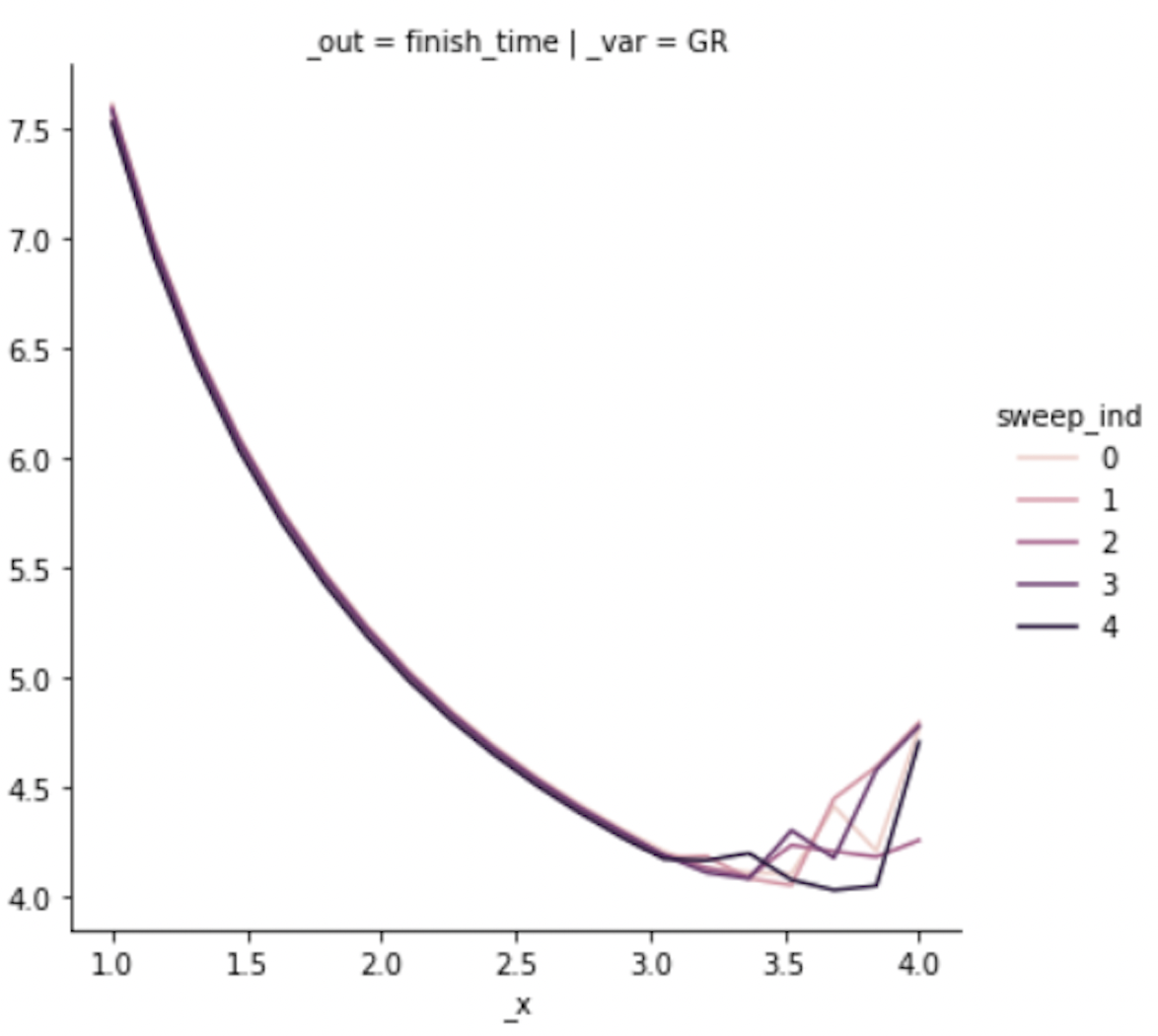
The following is the full version of the original student plot.
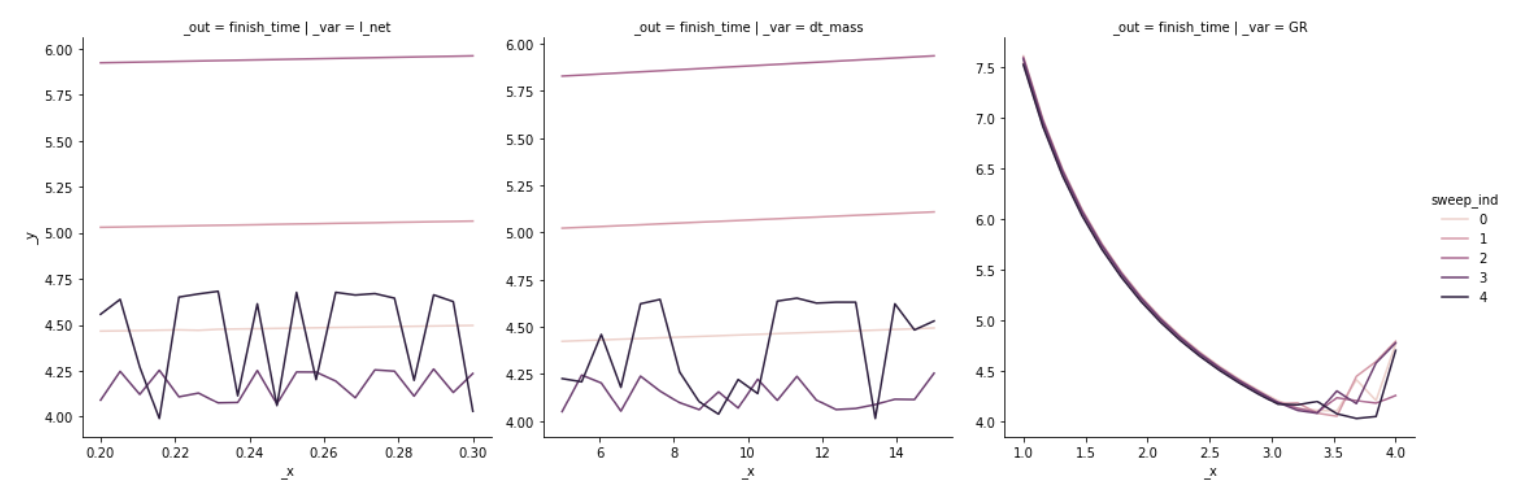